May 15 - May 19, 2017
Venue: Lipschitz lecture hall, Mathematics Center, Endenicher Allee 60, 53115 Bonn
Organisers: Seyedehsomayeh Hosseini, Boris Mordukhovich, André Uschmajew
Description: As many problems in computer vision, robotics, signal processing and geometric mechanics are expressed as nonsmooth optimization problems, the huge impact of efficient methods to solve these problems is undeniable. Even solving difficult smooth problems sometimes leads to the use of nonsmooth optimization methods to make the problem either smaller in dimension or simpler in structure. It also may happen that a problem is analytically smooth, but numerically nonsmooth. Therefore, it is of eminent interest to develop useful computational and theoretical tools in these situations as well.
This workshop on nonsmooth optimization techniques and their applications will bring together leading experts from different research groups from all over the world to present the latest theoretical and practical achievements in this growing field, fostering collaboration and discussing future developments. Topics include, but are not limited to nonsmooth and variational analysis, optimization methods and their convergence analysis, applications in image processing, machine learning, etc.
In case of questions regarding this workshop, please contact noa2017(at)hcm.uni-bonn.de.
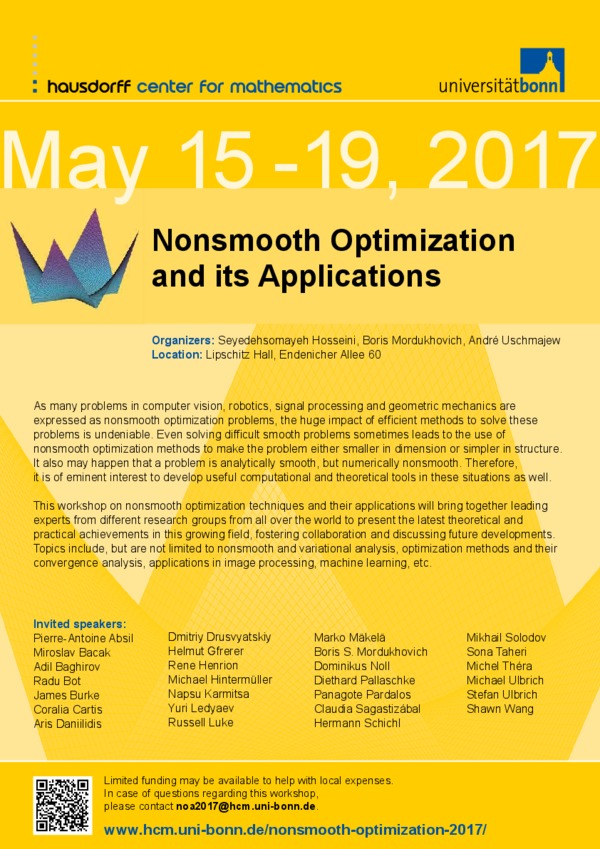
Participants
Name | Affiliation |
Pierre Antoine Absil | UC Louvain |
Lukas Adam | Czech Academy of Sciences |
Miroslav Bacak | Max Planck Institute for Mathematics in the Sciences |
Adil Baghirov | Federation University Australia |
Ali Barani | Lorestan University |
Debangana Baruah | University of Turku |
Radu Ioan Bot | University of Vienna |
Lynn Brandon | Springer Nature |
James Burke | University of Washington |
Coralia Cartis | University of Oxford |
Aris Daniilidis | Universidad de Chile |
Max Demenkov | Institute of Control Sciences Moscow |
Alireza Doagooei | Shahid Bahonar University of Kerman |
Achi Dosanjh | Springer Nature |
Dmitriy Drusvyatskiy | University of Washington |
Helmut Gfrerer | Johannes Kepler University (JKU) Linz |
Lisa Hegerhorst | Leibniz Universität |
René Henrion | Weierstrass Institute Berlin |
Michael Hintermueller | Weierstrass-Institut Berlin |
Benjamin Horn | TU Darmstadt |
Kaisa Joki | University of Turku |
Napsu Karmitsa | University of Turku |
Yuri Ledyaev | Western Michigan University |
Russell Luke | Universität Göttingen |
Boris Mordukhovich | Wayne State University |
Marko M. Mäkelä | University of Turku |
Ira Neitzel | Universität Bonn |
Dominikus Noll | Université Paul Sabatier |
Nina Ovcharova | Universität der Bundeswehr München |
Diethard Pallaschke | Karlsruhe Institute of Technology (KIT) |
Max Pfeffer | MPI MIS Leipzig |
Claudia Sagastizábal | IMPA |
David Salas | Université de Perpignan |
Hermann Schichl | Universität Wien |
Bishwesvar Singh | University of Turku |
Vinay Singh | National Institute of Technology Mizoram |
Mikhail Solodov | IMPA |
Sona Taheri | Federation University |
Michel Thera | University of Limoges |
Michael Ulbrich | Technische Universität München |
Stefan Ulbrich | Technische Universität Darmstadt |
Anna Walter | TU Darmstadt |
Xianfu Wang | University of British Columbia |
Schedule
Monday, May 15
08:30 - 09:00
Self-registration
09:00 - 09:20
Opening
09:20 - 10:00
Michael Hintermüller: (Pre)Dualization, Dense Embeddings of Convex Sets, and Applications in Image Processing
For a class of non smooth minimization problems in Banach spaces, predualization results and their connection to dense embeddings of convex sets are discussed. Motivating applications are related to nonsmooth filters in mathematical image processing. For this problem class also some numerical aspects are highlighted including primal/dual splitting or ADMM-type methods as well as proper (numerical) dissipation reducing discretization.
10:00 - 10:40
Yuri Ledyaev: Multidirectional mean-value inequalities and dynamic optimization
We discuss recent results on generalized mean-value inequalities for lower semicontinuous functions for smooth Banach spaces. Previously, such inequlities have been used to establish relations between Dini derivatives and subgradients of lower semicontinuous functions, to derive metric regularity of functional relations or equivalence of viscosity and minimax solutions in theory of generalized solutions of first-order PDE. In this talk we demonstrate how new mean-value inequality can be used to provide shorter proofs of optimality conditions for nonsmooth calculus of variation problems. We also discuss application of these results to nonsmooth dynamic optimization problems on manifolds.
10:40 - 11:10
Coffee Break
11:10 - 11:50
Adil Baghirov: Nonsmooth DC Optimization: Methods and Applications
An unconstrained optimization problem with the objective function represented, implicitly or explicitly, as a difference of two convex functions is considered. Numerical methods for solving such problems are designed and their convergence is discussed. Applications of these methods in machine learning and regression analysis are also considered.
11:50 - 12:30
Helmut Gfrerer: On first-order optimality conditions for general optimization problems
The basic first-order optimality condition states that the negative gradient of the objective belongs to the regular normal cone of the feasible region. In the nonconvex case the computation of the regular normal cone is a nontrivial task, and therefore one has to confine himself with some estimates of it. It is easy to find a lower estimate of the regular normal cone yielding so-called S-stationarity conditions. For an upper estimate one often uses the limiting normal cone which results in so-called M-stationarity conditions. Though a comprehensive calculus is available for computing limiting normals, sometimes it is very difficult or even impossible to give a point-based representationof the limiting normal cone. In this talk we provide a tighter estimate for the regular normal cone by means of regular normals to a series on tangent cones to tangent cones of the feasible set. We present an example of a mathematical program with equilibrium constraints, where this approach yields manageable optimality conditions wheras it is impossible to write down the M-stationarity conditions.
12:30 - 14:30
Lunch Break
14:30 - 15:10
James Burke: Variational Analysis of Convexly Generated Spectral Max Functions
The spectral abscissa is the largest real part of an eigenvalue of a matrix and the spectral radius is the largest modulus. Both are examples of spectral max functions---the maximum of a real-valued function over the spectrum of a matrix. These mappings arise in the control and stabilization of dynamical systems. In 2001, Burke and Overton characterized the regular subdifferential of the spectral abscissa and showed that the spectral abscissa is subdifferentially regular in the sense of Clarke if and only if all active eigenvalues are nonderogatory (geometric multiplicity of 1). In this talk we discuss how the same result can be obtained for the class of convexly generated spectral max functions.
15:10 - 15:50
Lukas Adam: Chance constrained problems: A view from variational analysis
We consider problems with joint chance constraints where the data are nonlinear, possibly nonconvex, random functions with finite distribution. Such problems are generally hard to tackle as the feasible set is a union of certain sets. We introduce integer variables and by their relaxation we arrive at an optimization problem with a MPCC-like structure. We derive strong stationarity conditions for this problem and relate them to the stationarity conditions of the original problem. Based on MPCC regularizations, we propose an iterative algorithm and show its convergence.
15:50 - 16:20
Coffee Break
16:20 - 17:00
Coralia Cartis: Universal regularization methods -- varying the smoothness, the power and the accuracy
Adaptive cubic regularization methods have emerged as a credible alternative to linesearch and trust-region for smooth nonconvex optimization, with optimal complexity amongst second-order methods. Here we consider a general/new class of adaptive regularization methods, that use first- or higher-order local Taylor models of the objective regularized by a(ny) power of the step size and applied to convexly-constrained optimization problems.
We investigate the worst-case evaluation complexity/global rate of convergence of these algorithms, when the level of sufficient smoothness of the objective may be unknown or may even be absent. We find that the methods accurately reflect in their complexity the degree of smoothness of the objective and satisfy increasingly better bounds with improving accuracy of the models. The bounds vary continuously and robustly with respect to the regularisation power, the accuracy of the model and the degree of smoothness of the objective. This work is joint with Nick Gould (Rutherford Appleton Laboratory, UK) and Philippe Toint (University of Namur, Belgium).
Tuesday, May 16
09:20 - 10:00
Pierre-Antoine Absil: Nonsmooth optimization on manifolds
Manifolds can be thought of as the mathematically adequate generalization of R^n as the search space of smooth optimization problems. However, there are also several important problems that can be phrased as the optimization of a nonsmooth function on a (smooth) manifold, e.g., sparse PCA, the optimal bounding box problem, some versions of the economic dispatch problem, and range-based independent component analysis. The purpose of this talk is to present some of these applications and to discuss algorithms available to address them.
10:00 - 10:40
Michael Ulbrich: A Proximal Gradient Method for Matrix Optimization Problems Arising in Ensemble Density Functional Theory
We present a proximal gradient method to solve a nonconvex optimization problem that is equivalent to the ensemble density functional theory (EDFT) model for electronic structure calculations in computational chemistry. The EDFT model is especially well suited for metallic systems. It can be cast as a matrix optimization problem with orthogonality constraints. Our algorithm uses an equivalent reformulation in which the singular values of the matrix-valued optimization variable arise in the cost function and the constraints. For the proposed proximal gradient method, convergence to stationary points is established. Numerical results show that this method can outperform the well-known self-consistent field iteration on many metallic systems.
10:40 - 11:10
Group photo and coffee break
11:10 - 11:50
Michel Thera: Perturbation of error bounds
In the current presentation, I intend to extend the developments in Kruger, Ngai and Thera, SIAM J. Optim. 20(6), 3280--3296 (2010) and, more precisely, to characterize, in the Banach space setting, the stability of the local and global error bound property of inequalities determined by proper lower semicontinuous under data perturbations. I will propose new concepts of (arbitrary, convex and linear) perturbations of the given function defining the system under consideration, which turn out to be a useful tool in our analysis. The characterizations of error bounds for families of perturbations can be interpreted as estimates of the `radius of error bounds'.
The definitions and characterizations are illustrated by examples. This presentation summarizes recent joint works with Huynh Van Ngai, Marco Lopez Cerda and A. Kruger.
11:50 - 12:30
Stefan Ulbrich: Optimization of elastoplastic contact problems with applicationto deep drawing processes
Hydroforming of sheet metals involves elastoplasticity and frictional contact, which can be modeled by an evolutionary quasi-variational inequality (EQVI). The aim is to control the blank holder force and the fluid pressure in such a way that no damages occur during the forming process and that the final shape of the sheet metal product coincides with the desired geometry. This leads to a challenging optimization problem for an EQVI given by a multiplicative finite plasticity model with frictional contact. We propose a semismooth FE-discretization of the EQVI. For the solution of the resulting reduced optimization problem we apply a trust-region bundle method and give some analytical justification for a simpler model problem. To deal with the high computational costs, we discuss model reduction techniques to reduce the complexity of the discretized EQVI. Moreover, we show optimization results for a hydroforming process based on the full FE-discretization and discuss a strategy towards an efficient reduced order model based optimization approach.
12:30 - 14:30
Lunch Break
14:30 - 15:10
Diethard Pallaschke: Quasidifferentiability and the Minkowski-Radström-Hörmander Lattice
For a finite-dimensional linear space X the quasi differential calculus of V.F. Demyanov and A.M. Rubinov has some similarities to the classical Frechet differential calculus. More exactly, in the quasi differential calculus the dual space X′ of gradients from the Frechet calculus is replaced by the Minkowski-Radström-Hörmander Lattice MRH(X) of differences of sublinear functions. First we show that the space MRH(X) can be endowed with a norm under which it becomes a Banach-space. Since the elements of Minkowski-Radström-Hörmander Lattice consists of equivalence classes and have no unique representation we study two types of minimal representatives, namely reduced and inclusion minimal representatives. Finally we consider the topological dual of MRH(X) and give for the 2-dimensional case an explicit example of a norm continuous linear functional, which is not continuous with respect to the supremum norm.
15:10 - 15:50
Miroslav Bacak: On proximal mappings and their generalizations
Proximal mappings were introduced by Moreau in the 1960's. Since then, they have become an indispensable tool in convex analysis and optimization in Hilbert spaces. In this talk I will present possible generalizations of proximal mappings outside of Hilbert spaces.
15:50 - 16:20
Coffee Break
16:20 - 17:00
David Salas: Characterizations of nonconvex sets with smooth boundary in terms of the metric projection in Hilbert spaces
The present communication is a continuation of a previous work over conditions for regularity of the metric projection. Here we provide a full characterization of closed bodies in Hilbert spaces with C^{p+1}- smooth boundary in terms of the smoothness of the metric projection and the behavior of its first derivative. The smoothness of the metric projection onto C^{p+1}-submanifolds of Hilbert spaces is also studied. Finally, we apply our results to the study of solutions of projected differential equations.